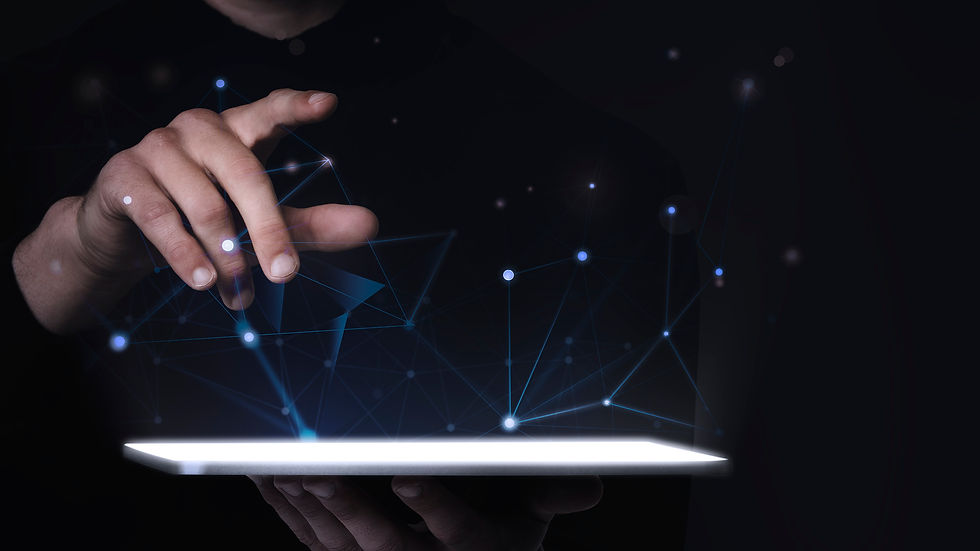
Generative AI and Predictive AI are two powerful technologies making waves in the finance sector. Understanding their unique characteristics, data requirements, impacts, benefits, and challenges is crucial for professionals looking to leverage these tools effectively.
Generative AI focuses on creating new content or data by employing models like Generative Adversarial Networks (GANs) and Variational Autoencoders (VAEs). These models can generate realistic and novel outputs, such as synthetic fraud scenarios, personalized financial strategies, and risk models that mimic real-world data. On the other hand, Predictive AI aims to forecast future outcomes based on historical data. It utilizes machine learning algorithms, including regression analysis, decision trees, and neural networks, to predict stock prices, market trends, and customer behaviors. This capability enables financial institutions to make informed decisions, optimize investments, and manage risks more effectively.
Both Generative and Predictive AI rely heavily on data, but their needs differ. Generative AI requires large volumes of diverse and high-quality data to learn the underlying patterns and nuances necessary for creating realistic outputs. This includes historical financial data, market reports, transaction records, and other relevant datasets. In contrast, Predictive AI depends on structured, clean, and labeled data to accurately forecast future events. In finance, this often involves historical price data, economic indicators, and consumer behavior data. Data quality is crucial; any inaccuracies or biases can significantly skew predictions.
The integration of Generative and Predictive AI into the finance sector offers numerous advantages. Enhanced risk management is one key benefit. Predictive AI models can identify potential risks by analyzing historical data and predicting future trends, allowing financial institutions to proactively manage risks, allocate capital more efficiently, and improve regulatory compliance. Additionally, Generative AI can simulate fraudulent activities, helping institutions recognize and respond to new fraud patterns. Predictive AI can analyze transaction data in real time to detect anomalies and prevent fraud.
Personalized financial services are another significant advantage. Both types of AI can tailor financial products to individual customers. Predictive AI can anticipate customer needs and preferences, while Generative AI can create personalized investment strategies and financial advice. Improved decision-making is yet another benefit. Predictive AI provides insights into market trends and economic conditions, enabling better investment decisions. Generative AI can model various financial scenarios, helping decision-makers evaluate potential outcomes and choose the best course of action.
Operational efficiency is also improved through AI integration. Automating routine tasks with AI reduces operational costs and improves efficiency. For example, AI can handle data entry, process transactions, and generate reports, allowing human employees to focus on more strategic activities.
Despite their benefits, the deployment of Generative and Predictive AI in finance comes with challenges. Handling large volumes of sensitive financial data raises concerns about privacy and security. Ensuring data protection and complying with regulations like GDPR and CCPA is critical. Additionally, AI models can inadvertently perpetuate biases present in the training data, leading to unfair treatment of certain customer groups and reputational damage. Continuous monitoring and bias mitigation strategies are essential.
The complexity and transparency of AI models pose another challenge. Deep learning algorithms, in particular, can be opaque, making it difficult to understand how decisions are made. This "black box" nature poses challenges for transparency and accountability. Integrating AI solutions into existing infrastructures can also be time-consuming and costly, especially for financial institutions relying on legacy systems.
The financial sector is highly regulated, and ensuring AI systems comply with all relevant laws and regulations is a significant challenge. Continuous updates to regulatory frameworks are needed to keep pace with technological advancements. To fully leverage the potential of Generative and Predictive AI, financial institutions must invest in data quality, prioritize security, address bias, enhance transparency, and stay compliant with regulations.
Generative and Predictive AI are poised to revolutionize the finance sector by enhancing risk management, improving decision-making, and personalizing financial services. By addressing the associated challenges, financial institutions can harness these technologies to drive innovation and achieve a competitive edge in an increasingly AI-driven world.
For more information on how your business can benefit from these cutting-edge AI solutions, contact Marketware today.
Comments